Machine Learning in Healthcare: The Future of Medical Innovation
Machine learning (ML) is rapidly transforming healthcare, ushering in an era where data-driven insights enable more accurate diagnoses, efficient treatment plans, and groundbreaking advancements in drug discovery. This article will explore the current state of machine learning in healthcare, the key benefits of adopting this technology, real-world products, and the most efficient ways to acquire these solutions. By diving deep into the use cases, product comparisons, and practical benefits, we will outline why machine learning is essential for the future of medicine.
What is Machine Learning in Healthcare?
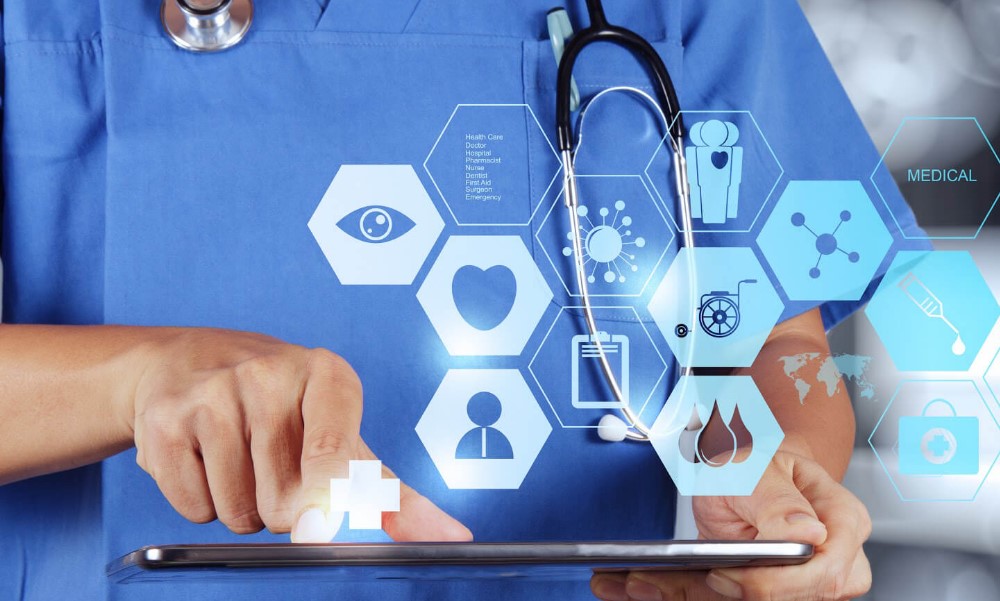
At its core, machine learning in healthcare is the application of AI algorithms to analyze massive amounts of health data—ranging from medical imaging to electronic health records (EHRs)—to provide valuable insights. These insights can help in identifying disease patterns, predicting patient outcomes, streamlining administrative tasks, and even discovering new treatments.
Machine learning algorithms are capable of learning from new data and improving their accuracy over time. They can be applied to a variety of tasks, including:
- Medical Imaging: Used to detect abnormalities in X-rays, MRIs, and CT scans.
- Predictive Analytics: Forecasting patient outcomes, including the likelihood of readmissions and disease outbreaks.
- Natural Language Processing (NLP): Analyzing clinical notes and EHRs to extract meaningful data.
- Genomics: Assisting in the identification of genetic risk factors for diseases.
- Clinical Trials: Optimizing patient recruitment and predicting trial outcomes.
Key Functions of Machine Learning in Healthcare
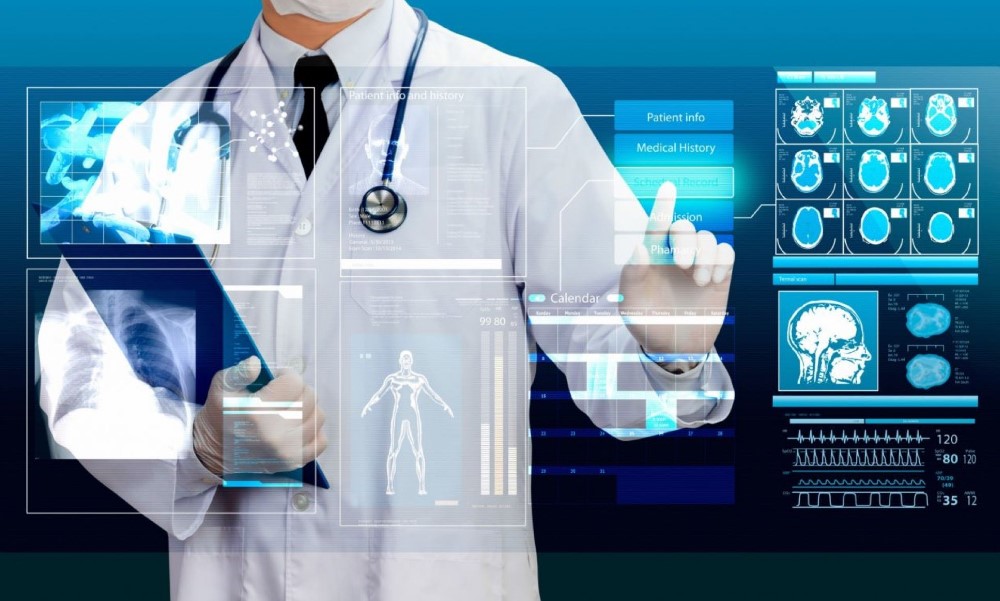
- Pattern Recognition: One of the key features of ML in healthcare is the ability to identify patterns within large datasets. Whether it’s recognizing a tumor in an MRI scan or predicting which patients are most likely to develop a certain condition, pattern recognition allows for faster and more precise diagnosis.
- Predictive Modeling: Predictive modeling uses past data to forecast future outcomes. For example, machine learning models can predict which patients are most likely to develop a chronic disease, enabling early intervention and preventive care.
- Automation: By automating repetitive tasks such as medical image analysis or administrative work, machine learning frees up healthcare professionals to focus on more complex tasks, improving overall efficiency in the healthcare system.
- Personalization: Machine learning algorithms enable highly personalized treatment plans by analyzing individual patient data. This helps healthcare providers deliver more effective care tailored to each patient’s unique needs.
Benefits of Machine Learning in Healthcare
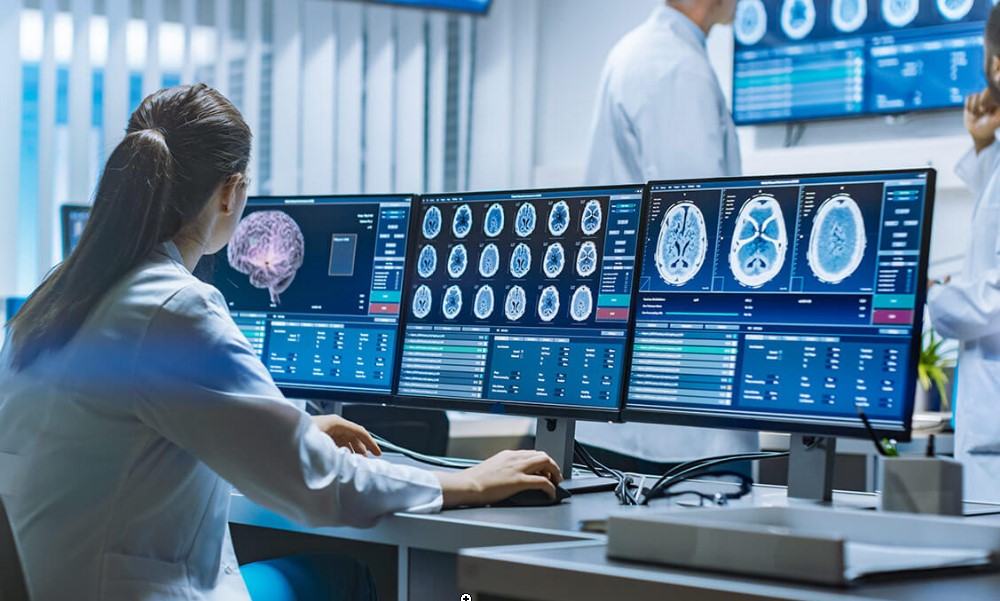
The integration of machine learning into healthcare has brought about a multitude of benefits. Let’s explore some of the most significant advantages in more detail:
1. Enhanced Diagnostic Accuracy
Machine learning can help reduce diagnostic errors by analyzing vast amounts of data to identify disease patterns that might be missed by the human eye. In areas like radiology and pathology, machine learning tools have demonstrated the ability to detect cancers, heart diseases, and other conditions earlier and more accurately than traditional diagnostic methods.
- Example: AI models can analyze radiological images for early detection of conditions like lung cancer or pneumonia. These models can flag abnormalities and prioritize high-risk cases for review, potentially saving lives by ensuring timely treatment.
2. Predictive Analytics for Early Intervention
Machine learning enables healthcare providers to predict patient outcomes based on historical data, enabling early interventions that can prevent complications and reduce hospital readmissions.
- Example: Predictive analytics can help identify which patients are at higher risk of developing chronic conditions like diabetes or heart disease, allowing physicians to develop preventive treatment plans tailored to those individuals.
3. Personalized Medicine
Personalized medicine tailors medical treatments to the individual characteristics of each patient, such as their genetic profile or medical history. Machine learning algorithms analyze genetic data to identify which treatments will be most effective for specific patients.
- Example: In oncology, personalized treatment plans are developed based on a patient’s genetic mutations. Machine learning models can recommend targeted therapies, increasing the chances of treatment success.
4. Operational Efficiency
By automating tasks such as appointment scheduling, billing, and claim processing, machine learning can greatly reduce the administrative burden on healthcare providers. This leads to more efficient operations, enabling staff to focus more on patient care.
- Example: Machine learning-powered chatbots can assist patients in scheduling appointments or answering common medical questions, freeing up administrative staff for more complex tasks.
5. Drug Discovery and Development
Machine learning accelerates the drug discovery process by identifying promising drug candidates, simulating their interactions, and predicting their effects on different patient groups. This drastically reduces the time and cost involved in bringing new drugs to market.
- Example: AI models have been used in the development of COVID-19 vaccines, helping scientists predict which molecular structures would trigger the best immune responses.
Real-World Machine Learning Products in Healthcare
Now, let’s explore five real-world machine learning products that are revolutionizing the healthcare industry. Each product addresses different use cases and provides cutting-edge solutions for both healthcare providers and patients.
1. IBM Watson Health
IBM Watson Health is an advanced AI platform that helps healthcare providers analyze clinical data, improve diagnostics, and develop personalized treatment plans. By leveraging machine learning algorithms, Watson Health can provide insights that enhance patient outcomes and improve the efficiency of healthcare systems.
- Use Case: Oncology and radiology departments use IBM Watson Health to analyze patient data and develop treatment plans.
- Pros: Scalable for large healthcare institutions, integrates with multiple data sources.
- Cons: Expensive for smaller clinics, requires extensive integration support.
- Price: Pricing is custom, based on the specific needs of the healthcare institution.
- Features: Predictive analytics, personalized treatment recommendations, clinical decision support.
2. Google Health AI
Google Health AI focuses on applying machine learning to medical imaging, particularly in fields like radiology and pathology. Its AI algorithms can identify abnormalities in imaging results and assist healthcare professionals in diagnosing conditions more accurately.
- Use Case: Radiology departments use Google Health AI for real-time imaging analysis.
- Pros: High accuracy, fast data processing, excellent for large datasets.
- Cons: Limited specialty focus, potential data privacy concerns.
- Price: Subscription-based; pricing is determined by usage and the specific solution package.
- Features: Real-time analysis of medical images, predictive diagnostics, deep learning models for disease detection.
3. PathAI
PathAI is a machine learning platform designed to assist pathologists in diagnosing diseases more accurately. The tool uses deep learning algorithms to detect patterns in pathology slides, which can lead to quicker and more accurate diagnoses of conditions such as cancer.
- Use Case: Used by pathologists to improve diagnostic accuracy in detecting cancerous cells.
- Pros: Increases diagnostic accuracy, reduces human error.
- Cons: Limited to pathology, requires trained professionals to interpret results.
- Price: Subscription-based, customizable depending on the size and needs of the institution.
- Features: AI-powered pathology insights, image-based diagnostics, real-time collaboration.
4. Aidoc
Aidoc is an AI-driven platform that specializes in the real-time analysis of medical imaging. Designed for use in emergency departments, Aidoc provides real-time alerts for critical conditions, allowing doctors to prioritize urgent cases quickly and effectively.
- Use Case: Emergency rooms use Aidoc to analyze medical imaging results and identify life-threatening conditions such as strokes or brain hemorrhages.
- Pros: Real-time alerts, critical care prioritization, fast data processing.
- Cons: Limited focus on imaging, primarily geared towards emergency settings.
- Price: Tiered pricing based on hospital size and the number of scans processed.
- Features: AI-powered diagnostics, PACS system integration, real-time alerts.
5. Zebra Medical Vision
Zebra Medical Vision provides machine learning tools for analyzing medical imaging data. Their platform is used to detect diseases like cancer, liver conditions, and cardiovascular problems. Zebra’s AI tools continuously learn and improve, helping radiologists make faster and more accurate diagnoses.
- Use Case: Radiology departments use Zebra Medical Vision to scan medical images and detect signs of serious diseases.
- Pros: Comprehensive disease detection, regular updates to algorithms, cost-effective for large institutions.
- Cons: Primarily focused on imaging data, requires substantial training.
- Price: SaaS (Software-as-a-Service) model with per-scan pricing.
- Features: Multi-modality image analysis, AI-powered disease detection, cloud-based infrastructure.
Product Comparison Table
Product | Use Case | Pros | Cons | Price | Key Features |
---|---|---|---|---|---|
IBM Watson Health | Oncology, personalized care | Highly scalable, multi-source integration | Expensive, requires expertise | Custom pricing | Predictive analytics, personalized treatment |
Google Health AI | Radiology imaging | Fast, highly accurate analysis | Data privacy concerns | Subscription | Real-time imaging, disease detection |
PathAI | Pathology diagnostics | Increases diagnostic accuracy | Requires expert interpretation | Subscription | AI-driven pathology insights |
Aidoc | Emergency care imaging | Real-time alerts, critical care | Limited to imaging | Tiered pricing | AI triage, fast alerts |
Zebra Medical Vision | Disease detection imaging | Wide range of disease detection | Imaging data only | Per-scan pricing | Multi-modality image analysis |
Why Machine Learning Products are Crucial in Healthcare
Machine learning tools are no longer just supplementary but are now essential in healthcare. They improve diagnostic accuracy, reduce errors, increase efficiency, and personalize treatments in ways that human-only methods cannot match. Below are some detailed use cases for machine learning products in healthcare:
1. Radiology
Machine learning can analyze X-rays, CT scans, and MRIs faster and more accurately than human radiologists. Products like Google Health AI and Zebra Medical Vision are specifically designed to improve accuracy in identifying fractures, tumors, and other anomalies. By speeding up the diagnostic process, patients receive faster treatment, which can be critical for conditions like cancer.
2. Personalized Medicine
IBM Watson Health excels in this area, using machine learning to analyze genetic data and recommend personalized treatment plans. This is especially valuable in oncology, where targeted therapies can make a significant difference in patient outcomes.
3. Emergency Care
Products like Aidoc enable hospitals to prioritize care based on real-time imaging data. In a crowded emergency room, this can be the difference between life and death. Aidoc’s machine learning algorithms analyze imaging results and flag critical cases for immediate review, improving the speed of emergency interventions.
How and Where to Buy Machine Learning Products
Purchasing machine learning products for healthcare institutions typically involves direct consultation with vendors. Below is a guide to purchasing some of the top machine learning solutions:
- IBM Watson Health
Purchase here. IBM offers custom pricing based on the scale and specific needs of your healthcare institution. They provide demo consultations to help identify the right solutions for your needs. - Google Health AI
Purchase here. Google Health operates on a subscription basis, and you can contact them directly to request a quote based on your institution’s needs. Google Health AI is suitable for large-scale radiology departments and academic medical centers. - PathAI
Purchase here. PathAI offers subscription-based pricing with customized plans for different healthcare facilities. Contact their sales team for a demo and pricing information. - Aidoc
Purchase here. Aidoc uses tiered pricing based on the number of scans processed. It’s ideal for emergency departments in large hospitals and trauma centers. - Zebra Medical Vision
Purchase here. Zebra Medical Vision uses a per-scan pricing model, making it a cost-effective solution for institutions that process a large volume of imaging data.
Frequently Asked Questions (FAQ)
1. How does machine learning improve diagnostic accuracy?
Machine learning algorithms can process large amounts of data quickly and identify patterns that may not be easily detected by the human eye, leading to earlier and more accurate diagnoses.
2. Are machine learning tools affordable for small clinics?
Some machine learning products can be costly, especially for smaller clinics. However, solutions like Zebra Medical Vision offer per-scan pricing, making it more accessible for smaller facilities.
3. Is patient data safe when using machine learning tools?
Most machine learning products in healthcare are designed with strict data privacy and security measures to protect patient information, but institutions must ensure compliance with relevant privacy regulations like HIPAA.
4. How do healthcare providers integrate machine learning tools?
Most machine learning solutions offer integrations with existing healthcare IT systems like EHRs and PACS, although the process may require specialized technical support.
5. Can machine learning be used for preventive care?
Yes, machine learning algorithms can predict the likelihood of developing certain conditions, allowing for early intervention and preventive treatment plans.